Announcement
- Validation Submission Re-opened!! (January 21, 2021)
We highly appreciate your continued interest in PAIP 2020 Challenge.
Validation submission is reopened and you can check the rank and F-1 score on the Validation Leaderboard.
If you have inquiries, please contact us at paip.challenge@gmail.com
Schedule (2020)
- Challenge start and release of training dataset (April 14)
- Evaluation metrics release (April 25)
- Release of submission rules for validation phase (May 06)
- Validation dataset release and start of validation submission (August 13)
- Team merger deadline (August 31)
- End of validation phase (August 31)
- Test dataset release and start of final submission (September 03)
- End of submission (September 15)
- Top10 notification and invitation for workshop (October 8)
- Challenge Workshop in conjunction with virtual KOSOMBE autumn conference (November 12)
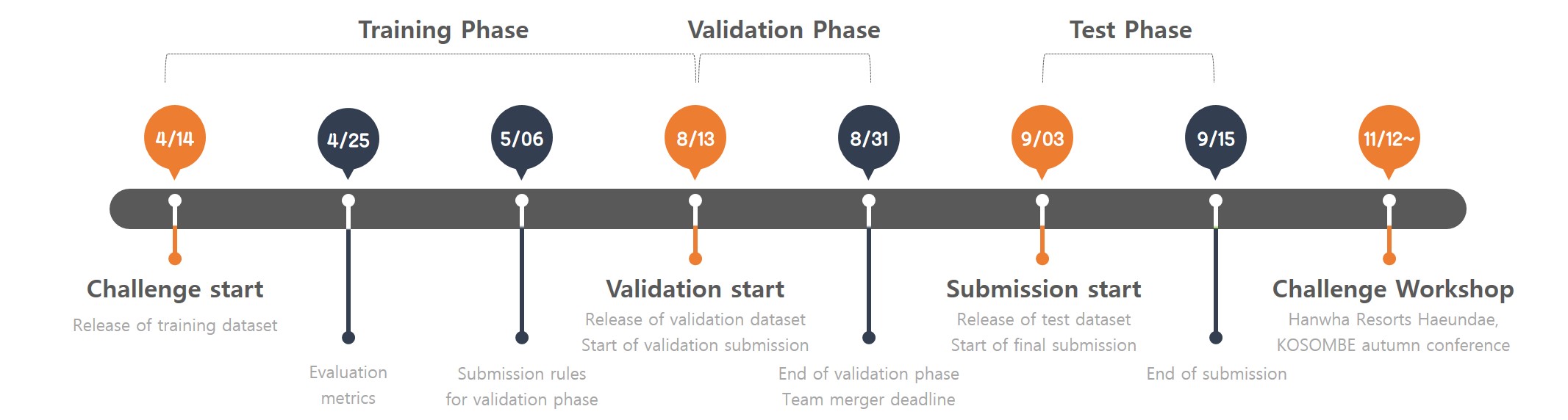
Motivation
Welcome to the PAIP2020 Challenge website!
Built on the success of its predecessor, PAIP2020 is the second challenge organized by the Pathology AI Platform (PAIP) and the Seoul National University Hospital (SNUH). On this page, you can register for the challenge, download dataset, and submit your results.
Last year at the MICCAI 2019, we organized the successful PAIP2019 grand challenge, and received 64 submissions from 28 research groups. The PAIP2019 challenge was based on a slide level image analyses to segment whole tumor areas in liver cancer and calculate their viable burden using whole-slide images (WSIs). This year, PAIP2020 will proceed to not only detect whole tumor areas in colorectal cancers but also to classify their molecular subtypes, which will lead to characterization of their heterogeneity with respect to prognoses and therapeutic responses.
Aims
The goal of the PAIP2020 challenge is to evaluate new and existing algorithms for the automated classification of molecular subtypes in colorectal cancer for whole-slide image analyses. As the task, all participants should predict one of the molecular carcinogenesis pathways, i.e., microsatellite instability(MSI) in colorectal cancer, by performing digital image analysis without clinical tests. This task has a high clinical relevance as the currently used procedure requires an extensive microscopic assessment by pathologists. Therefore, those automated algorithms would reduce the workload of pathologists as a diagnostic assistance.
TASK : MSI-High Classification in Colorectal Cancer
Notification ! : The MSI-High(MSI-H) classification is the main task, which has first priority consideration in the challenge evaluation, while segmentation is a mandatory task for this challenge. Therefore, all participants who want to make a submission need to enclose the result of the MSI-H prediction and segmentation of the whole tumor area.
Background
Colorectal cancer (CRC) is an alarming threat to health in terms of cancer-related deaths in developed and developing countries. Statistically, CRC is the third-most commonly diagnosed malignancy in the United States and South Korea. CRC develops as a result of the accumulation of genetic and epigenetic alterations and is known as a heterogeneous disease entity with three molecular carcinogenesis pathways and two morphologic multistep pathways. The molecular subtypes of CRC exhibit different responses to adjuvant therapy, which may be responsible for differences in subtype-specific survival. Three molecular carcinogenesis pathways have been identified: (1) chromosomal instability (CIN), (2) microsatellite instability (MSI), and (3) CpG island methylator phenotype (CIMP) or epigenetic instability pathways. In this challenge, we will focus on MSI detecting in cases of CRC.
MSI is a condition of genome-wide alterations in the number of repeat nucleotide(s) caused by a defective DNA mismatch repair by epigenetic inactivation of MLH1 gene or germline mutations in mismatch repair genes. The microsatellite status of each tumor was determined by the evaluation of five microsatellite markers: D2S123, D5S346, D17S250, BAT25, and BAT26. The MSI status was classified as follows:
- MSI-High (MSI-H; instability at ≥ 2 microsatellite markers)
- MSI-Low (MSI-L; instability at 1 marker)
- MSI-Stable (MSS; microsatellite stable or no instability)
MSI-H in CRCs is significantly associated with a better prognosis but does not show benefit from 5-fluorouracil-based adjuvant chemotherapy. Moreover, recent evidence has indicated that MSI-H is a significant predictor of a positive response to immunotherapy using an immune checkpoint blockade in solid tumors. Therefore, the pathological reporting of MSI status is strongly recommended for all surgically resected CRC cases. In clinical practice, however, not every patient is tested for MSI because this requires additional genetic or immunohistochemical tests. By developing these MSI-H classification algorithms, prognoses and responses to immune checkpoint blockade therapy can be predicted simply by using digitally scanned slide images without additional time and cost required for the evaluation of microsatellite markers.
Dataset
In the challenge, participants will be provided with data sets of the whole tumor area level extracted from Whole Slide Image, and the MSI-H status information.
The data and segmentation of the entire tumor area are provided by the Seoul National University Hospital, South Korea. These are fully annotated by expert pathologists and are divided into three groups of data sets.
- The training dataset contains 47 WSIs
- The validation dataset contains 31 WSIs
- The test dataset contains 40 WSIs
All WSIs were scanned at 40X magnification and all cases are randomly selected irrespective of the participating institutions.
The ground truth information is given to participants for the training set. For the validation and test set, however, the ground truth information is reserved to the challenge committee and will be used to evaluate the performance of the AI learning models of each participant. (See the Detailed Data Description)
How to Participate
1. Read the rules of the challenge carefully.
2. Register at grand-challenge.org.
3. Join the PAIP2020 challenge.
4. Fill the Data Use Agreement Consent form.
5. Download the dataset from a confirmed email with link and access credentials.
6. Submit your result and manuscript abstract.
If you have questions or comments, post a message on the forum. We usually respond to the questions within seven days. However, if you need any immediate assistance, please contact us at paip.challenge@gmail.com, so that we can resolve any issues within 2-3 business days.
References
1. Singh, Manish Pratap, et al. "Molecular subtypes of colorectal cancer: an emerging therapeutic opportunity for personalized medicine." Genes & Diseases (2019).
2. Bae, Jeong Mo, Jung Ho Kim, and Gyeong Hoon Kang. "Molecular subtypes of colorectal cancer and their clinicopathologic features, with an emphasis on the serrated neoplasia pathway." Archives of pathology & laboratory medicine 140.5 (2016): 406-412.
3. Kim, Baek-hui, et al. "Standardized Pathology Report for Colorectal Cancer." Journal of pathology and translational medicine 54.1 (2020): 1.
4. Bae, Jeong Mo, et al. "Fibroblast growth factor receptor 1 (FGFR1) amplification detected by droplet digital polymerase chain reaction (ddPCR) is a prognostic factor in colorectal cancers." Cancer research and treatment: official journal of Korean Cancer Association 52.1 (2020): 74.
5. Kather, Jakob Nikolas, et al. "Deep learning can predict microsatellite instability directly from histology in gastrointestinal cancer." Nature medicine 25.7 (2019): 1054-1056.
Organizers






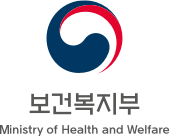
PAIP platform
PAIP provides more high-quality pathologic slide images of six tumor types as well as region-of-interest annotations developed by pathology experts. Please find this website http://wisepaip.org.